Welcome to this executive briefing on Natural Language Processing (NLP).
I appreciate that your time is valuable, and that’s why in this course, we will only cover the ultimate essentials for you to get up to speed with natural language processing and see tangible examples of how this technology can add substantial value to your business.
Here’s how this course is going to be structured:
- First, we will talk about what natural language processing is and why it has such a high potential to disrupt virtually any business and any industry
- Then we will discuss how natural language processing works and we will find out about the two components of NLP, natural language understanding and natural language generation
- Next, we will dive into what possible applications of natural language processing exist out there to help you come up with ideas of how this exponential technology could potentially be applied in your industry, in your business, then we will stop on one application specifically, and this application is Chadbourne. You’ve probably heard of Chad was disrupting how companies do customer service in this material
- Finally, we will look at 10 different case studies of natural language processing already being applied in different companies and industries
Here we are after the real results and the numbers so we can get a feel for what natural language processing can do for our businesses.
So, I hope you’re as excited as I am.
And without further ado, let’s dive into the world of natural language processing (nlp).
Table of Contents
- What is Natural Language Processing OR What is NLP?
- How does NLP works?
- Applications of NLP
- The Future of AI ChatBots
- 10 Use-cases – with Real Results
- Summary
What is Natural Language Processing OR What is NLP?
Natural language processing is the field of artificial intelligence concerned with the interactions between computers and human natural languages, in particular how to program computers to process and analyse large amounts of natural language data.
But before we dive into NLP, we need to understand the two types of data that exist structure, data and unstructured data.
- Structured data is data that has a predefined specific format, for example, spreadsheets, tables and databases.
- Unstructured data, on the other hand, is data that doesn’t follow a predefined specific format
For example, emails, chats, blogs, books, audio files, video files and images.
We humans communicate predominantly in unstructured data, specifically via natural language, and that’s why, based on various sources, unstructured data accounts for between 70 percent to 90 percent of data in the world. Most of this data contains text in some form, whether written or audio. This data, like all data, contains power, power to know your customers better, power to increase efficiency, power to scale.
However, because of its unstructured format, it is much harder to leverage, and that’s why natural language processing is an exploding field.
Right now, businesses are in a race to be the first in their industry to unlock the potential of their unstructured data, and in the following tutorials, we will explore what opportunities might exist in your business to leverage an LP.
How does NLP works?
There are two parts to NLP:
- Natural language understanding (NLU)
- Natural language generation (NLG)
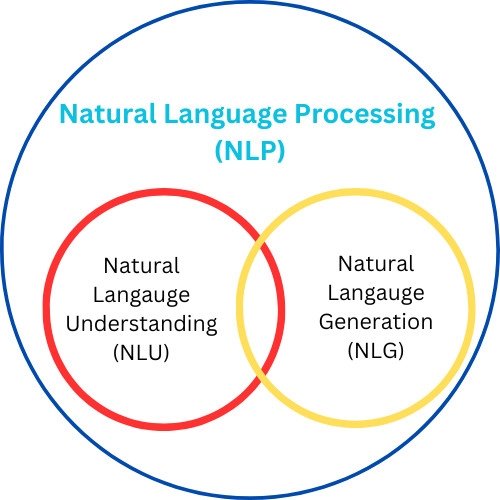
Natural language understanding refers to mapping the given input from natural language into formal representation and analyzing it.
If the input is in the form of audio, then speech recognition is applied first to convert it into text, and then the hard part starts the interpreting of the meaning from the text. Human language is complex.
Some words, for example, bank or leaves can carry different meaning depending on the context they are in. This is where advanced machine learning models such as Google’s State of the Art BERT come into interpret the meaning.
We’re not going to go into the technical details of this algorithms.
However, one thing to note here is that originally these algorithms were actually hard based rules like if. However, now they’re predominantly statistical models that calculate the probabilities of what a word or sentence should mean, thereby the use context of the whole sentence to understand the meaning
Once the meaning is extracted, it is categorized and the input is classified, and then we can come up with an appropriate action or response.
This is where NLG or natural language generation may be required, and algae is a process of producing meaningful phrases and sentences in the form of natural language from some internal representation, and algae is generally much easier than Anello, since when we know the meaning that needs to be expressed, there are certain rules of language such as syntax and semantics that need to be followed in order to create an appropriate sentence.
If the text needs to be put into audio like in the case of Siri or Alexa, then speech generation comes in as well.
But this is not always required, that’s in a nutshell, how NLP works.
The three important takeaways from this video are that an
- NLP has two parts NLU and NLG
- Some applications will require only one or the other
- Some applications will require both
Speech recognition and speech generation are parts of an NLP, but they only come into play when audio is part of the equation.
Most of text data is not in audio form.
And finally, the most complex part of an LP is extracting meaning from natural language. However, state of the art models is getting extremely good at this, meaning that businesses can process more and more unstructured data.
Applications of NLP
Every industry can leverage unstructured data in many different ways.
In this section, we will look at some of the possible applications of natural language processing to help you innovate and come up with ideas for your business application:
- Sentiment analysis – Help you understand how users feel about your company or product
- Speech recognition – Convert audio into text for further processing application
- ChatBots – Provide 24/7, 365 days a year customer support application. ChatGPT has taken the internet by storm as people are both fascinated and concerned at how intelligent the AI-powered bot sounds.
- Machine translation – Help you reach new markets with minimal investment application
- Completing text similar to what Gmail does – Help you increase your employee’s efficiency through a centralized knowledge base or to improve how your customers interact with your product or website application.
- Spell checking – It make sure that documents don’t contain errors, especially relevant in industries with high compliance requirements such as banking, insurance, and finance
- Keyword search – locate relevant information faster across all data facilities, increasing efficiency.
- Advertisement matching – For example, when you search for a new car on Google or write an email about it to a friend, you will get car ads, relevant to your search history
- Information extraction – Extract the essence from large volumes of unstructured data
- Spam detection – clean your email inbox
- Text generation – create new text, for example, client contracts, research documents, training materials and so on
- Automatic summarization – add summaries to existing documents
- Questions answering – answer specific questions based on disparate information sources
- Image captioning – annotate images and find any application number
- Video captioning – Videos can take a long time to watch. Sometimes it’s faster to extract information from them in the form of text and then process it further using other NLP techniques like summarization
So we looked at 15 different applications of NLP.
There are many more, but hopefully this has already given you some ideas how you might be able to apply natural language processing in your business.
The Future of AI ChatBots
All NLP applications are growing quite fast.
For example, ChatGPT – The AI-powered bot has gained immense popularity on the internet, with people being captivated and apprehensive about its remarkable intelligence.
As a conversational AI chatbot, ChatGPT has garnered an enormous amount of attention this year, marking a revolutionary moment in the world of technology. Some experts predict that its advent will signal the demise of Google Search as we currently know it, and this product has already set off alarm bells at the search giant. It is noteworthy that within just 5 days of its public launch in November 2022, ChatGPT had already amassed over 1M users.
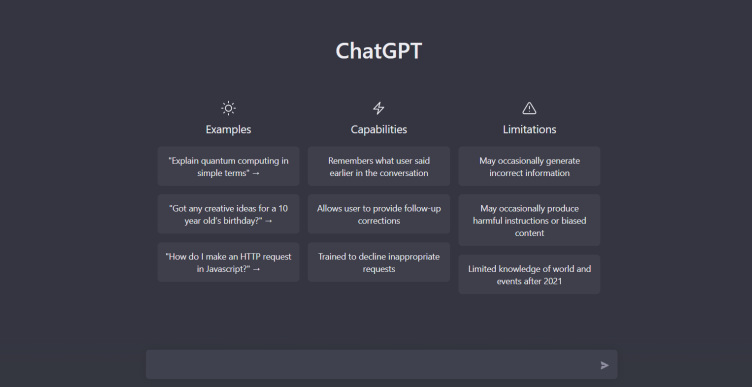
However, how does this figure compare with other major tech companies such as Netflix, Spotify, or Facebook? It took years/months to get their firs 1M users.
Over 250 billion customer support requests are made every year and it costs businesses an incredible 1.3 trillion dollars to service them. Most of these requests are simple and can be answered by as long as it has a deep knowledge base and is good enough at understanding natural language.
Moreover, this is what consumers want to, according to Live Person, which is a company that makes support software for Vodafone, IBM, Virgin Atlantic and others.
- At least 70% of consumers say that they prefer messaging over calling customer support
- According to Gartner, by 2020, 85 percent of all customer interactions will be handled without a human agent
What does this mean for companies adopting Chatbots?
Let’s look at some forecasts from Juniper Research:
HealthCare – The number of Chatbot interactions will grow from 21 million in 2018 to 2.8 billion by 2023
Retail – The number of successful Chatbot interactions will grow from 2.6 billion in 2019 to 22 billion in 2023
Banking – Operational cost savings from using Chatbot will grow from 209 million in 2019 to 7.3 billion in 2023. This represents time served for banks in 2023 of 862 million hours, equivalent to nearly half a million working years
Those are examples of cardboards in just three industries. Other examples include:
- Real estate agent bots
- Loan Calculator bots
- Life Coach bots
- Politician bots
- Investment Advice bots
- Home Service bots
- Appointment setting bots
- Employee onboarding bots and so on
The list goes on. Chat boards can and should be implemented in almost any industry.
So how do you get started?
Chatbot’s can be of varying complexity. However, predominately there are just two types
The simple and advanced
Simple chatbot’s use minimal natural language processing as they are pre coded to a certain set of questions and responses. These are very easy to set up.
For example, on Facebook messenger with tools like Minecart. This is already a great start because according to Pew Research, 69% of online adults in the United States are on Facebook.
Therefore, you have a strong probability that you can get your chatbox out in front of your target demographic. If you create a chat board for Facebook.
Advance Chatbots, heavily rely on natural language processing, specifically on the first part of it, natural language understanding or NLU.
They can deal with free form queries from customers and interact with them just as a human would. The good news is that you don’t need to invest into research in development here either. With tools like Watson assistant from IBM, you can get up and running within days.
For example, Autodesk uses Watson assistant to power its own Chatbot EVA. Here are some of the results they’ve seen so far.
- A 99% improvement in response times, cutting resolution time from 38 hours to just 5.4 minutes, for most tier one inquiries
- A drop in per query cost from $15 to $2 for human, agents to just $1 for virtual agents
- A virtual agent that answers and resolves an average of 30K+ customer support queries per month
- A virtual agent that recognizes 40+ distinct use cases to quickly resolve simple requests
So, there is an example of a chat bot in action and something that you could set up for your business as well.
10 Use-cases – with Real Results
In this section, we will look at 10 different examples of natural language processing (NLP) and action and how it is bringing value to businesses today.
So, let’s get started.
Rotterdam Airport
It has been used for document analysis.
Ports and other transport hubs are bustling centers of activity where everything is documented, but every document is a little bit different. Until recently, the variety of these documents was too much for computers to handle, and huge teams of people worked on analyzing incoming documents in various languages, formats and styles and logistics company in Rotterdam, Incorporated and LP program that parses documents and extracts product related information from them at 97 percent accuracy.
This reduced the time spent on processing documents by 98%, reduced shipping errors by 6% and saved $4M every year.
Autodesk and their Chabert Autodesk
Chatbots carries out over 1,00,000 customer conversations per month.
This allowed Autodesk to improve their response times by 99% from 38 hours to 5.4 minutes, replacing human support staff, which cost the company anywhere from $15 to $200 per query at the time they were used with. AWA also means that each query now costs less than a dollar.
Boston Consulting Group
They use NLP to analyze survey results.
Surveys are a great way for gathering valuable data, but only if that data can be properly gathered, understood and analyzed. This is often done manually due to the natural variety and length styles and even language of the responses.
Boston Consulting Group gathers survey responses from the 50 countries where its offices are situated, wanting to cut down on costs and time. They employed an algorithm to reduce the time necessary to process the results by 99.7%.
What used to take a team of 4 people a month now takes a team of 2 people just 2 hours.
JPMorgan Chase
Used natural language processing for document review.
Banks and other financial institutions need to operate with absolute clarity regarding their legal status. JPMorgan Chase used to employ an army of lawyers and loan officers to review legal documents amounting to 360000 hours of work annually.
Their new natural language processing system, called COIN, short for contract intelligence, does the
same work in seconds, providing huge savings and preventing clerical errors a leading cause of loan servicing mistakes.
YouTube
Processing Videos, recommending relevant videos to user and they’re considering mentoring system, the massive growth of content we’re experiencing isn’t purely positive.
There is also more and more disturbing, violent, or illegal content that needs to be prevented from spreading. YouTube, as well as other large social media networks have been battling this trend by employing ever larger numbers of human moderators.
But this is mostly seen as a temporary measure while technology catches up. In the first six months after YouTube started using an LP to analyze potentially violent and extremist content, the system reviewed and flagged content that would have taken one hundred and eighty thousand people working 40 hours a week to assess.
This not only saved the company millions of dollars and improved their reputation, it also spared people from viewing horrific content.
8Allocate
Uses an NLP for sentiment analysis.
Understanding how your customers feel about your brand is incredibly powerful and allows you to adjust your sales and marketing strategy. Also known as opinion mining sentiment analysis, algorithms use an LP to analyze social media news and blogs are marking it as either positive, negative, or neutral.
A large, unnamed European retailer looking to increase customer loyalty achieved a 30 percent revenue increase within 12 months of deploying such a solution.
Virtual personal assistants
Voice driven assistants are on the rise.
It’s predicted that by 2020 you’ll be having more conversations with such an assistant than with your spouse. Whether that’s speaks more about voice assistance, or our society is up for debate. But it’s undeniable that this is a market with huge potential.
The virtual personal assistant market will grow to over 15 billion dollars by 2021, with an estimated 1.8 billion users, and help is huge for this rapid growth, since being able to work with your virtual assistants without using your hands is the number one reason for adopting the technology. Other benefits mentioned by users are that spoken language feels more natural than typing and that children find it easy to use further showing the power of natural language processing.
Sky
Used an LP for customer feedback analysis.
Listening to customer feedback gives a company invaluable insight, allowing it to adapt to their wishes and needs with the goal of greater retention and lifetime value. But often a company might get so much feedback that it’s difficult or even impossible to analyze it with traditional means.
Sky developed a solution for analyzing customer feedback for an international game developer and publisher. With over 60 million registered players providing feedback in various forms across multiple platforms, Sky introduced an MLP system to process all the incoming data.
Successful integration of the system resulted in a 95 percent reduction in costs and time spent with significant improvements in the quality of the process data within months of deployment.
Lenddo Credit Assessment
NLP for credit assessment.
Evaluating a potential customers credit worthiness is a big part of what makes a bank successful or not.
In developed nations, banks can access a lot of reliable information, including credit scores, bank statements and employment status. But denying people in developing nations loans just because they don’t have that information available doesn’t only promote wealth inequality. It also means losing a huge potential market.
Lender uses an LP to analyze a customer’s social media, browsing history and geolocation to understand their behavior and relationships, factors that are highly indicative of their credit worthiness. This has helped banks and Michael lenders verify customers identities and assess risk, which allowed them to safely provide loans to five million lenders across 15 countries, reaching customers they traditionally wouldn’t have had access to.
Booking.com
How they used natural language processing for translations.
There are over 27 million rooms available on booking dotcom in 220 countries from Australia to Zimbabwe. 1.5 million rooms are reserved every night, and two-thirds of those reservations are done in languages other than English.
For this reason, booking.com needed to develop a powerful translation engine and currently covers over 40 languages with automatic Real-Time translations.
Booking.com system currently scores 3.9 out of 4 inadequacy and a 3.78 out of four fluency compared to, for example, 3.96 and 3.82 for human translators from English to German.
So there we go
Those were 10 use cases, 10 examples of natural language processing in action in varying industries. And hopefully that gave you some ideas of how you can use and help in your business.
I hope this inspires some ideas of how you can use natural language processing (NLP), learning in your industry and in your business.
Summary
So in a nutshell,
Natural Language Processing (NLP) is a subfield of Artificial Intelligence (AI) that focuses on enabling machines to understand, interpret, and generate human language. NLP is a rapidly growing field with a significant impact on society, and it has the potential to revolutionize the way we communicate with computers and each other.
The potential for NLP to scale in the future is enormous. As more data becomes available, NLP algorithms can be trained to become more accurate and sophisticated. Additionally, new advancements in machine learning and deep learning are continually improving the capabilities of NLP systems.
So, there we go.
I really hope that now you are much more comfortable and confident speaking about natural language processing (nlp), and more importantly, seeing how you can apply them in your industry and in your business.
Thank you for reading our blog, we hope you found the information provided helpful and informative. We invite you to follow and share this blog with your colleagues and friends if you found it useful.
Share your thoughts and ideas in the comments below. To get in touch with us, please send an email to dataspaceconsulting@gmail.com or contactus@dataspacein.com.
You can also visit our website – DataspaceAI