The role of AI in advancement of technology, data analysis and decision making has led to an explosion of data in recent years, and organizations are struggling to make sense of it all.
Data analysis has become a crucial function for businesses to gain insights and make informed decisions.
Artificial Intelligence Technology has revolutionized the way we approach decision-making. With the ability to analyze large amounts of data and identify patterns and insights, AI has the potential to greatly enhance the efficiency and accuracy of decision making.
However, the sheer volume and complexity of data can make it challenging to extract meaningful insights. Artificial Intelligence (AI) has emerged as a powerful tool to address these challenges, making data analysis more efficient and accurate.
With AI, organizations can unlock the full potential of their data, gain a competitive edge, and make better decisions by optimizing the decision-making process. This article will explore how organizations can leverage AI for advanced data analysis and the benefits it can bring to their operations.
Table of Contents
- Introduction
- The Basics of AI for Data Analysis and Decision Making
- Current Advancements in Industry with the integration of AI
- Applications of AI in Data Analysis
- Tools and Technologies
- Best Practices
- Challenges and Limitations
- Conclusion: The Future of AI in Data Analysis
Introduction
Data analysis is an essential part of modern business operations, and the increasing role of Artificial Intelligence (AI) in this field has opened up new possibilities for organizations.
AI-based data analysis can help companies to extract insights from vast amounts of data, make better decisions, and gain a competitive edge.
In this article, we will explore how organizations can leverage AI for data analysis and the benefits it can bring to their operations.
The Basics of AI for Data Analysis and Decision Making
The basics of AI for data analysis involve the use of machine learning and natural language processing techniques.
- Machine Learning: Understanding the fundamental concepts and techniques of machine learning, such as supervised and unsupervised learning, and how it can be used for data analysis.
- Natural Language Processing (NLP): Exploring the basics of NLP, including techniques for text mining, sentiment analysis, and language translation.
- Deep Learning: Understanding the concepts and techniques of deep learning, including neural networks and convolutional neural networks, and how they can be used for data analysis.
- Reinforcement Learning: Understanding the basics of reinforcement learning, including Q-learning and Markov decision processes, and how it can be used for decision-making in data analysis.
- Supervised and Unsupervised Learning: Understanding the difference between supervised and unsupervised learning, and when to use one over the other for data analysis.
- Data Preparation and Feature Engineering: Understanding the importance of data preparation, such as cleaning and preprocessing, and feature engineering, such as selecting and creating the right features for a model.
- Model Evaluation: Understanding the importance of evaluating a model’s performance and making adjustments as needed.
- Data bias and interpretability: Understanding the importance of avoiding data bias and interpretability issues when using AI for data analysis.
Current Advancements in Industry with the integration of AI
In the context of fraud detection, AI can be used to analyze transaction data, identify patterns of fraudulent activity, and flag suspicious transactions. It can also be used to identify patterns in customer behavior, such as changes in spending habits or account activity.
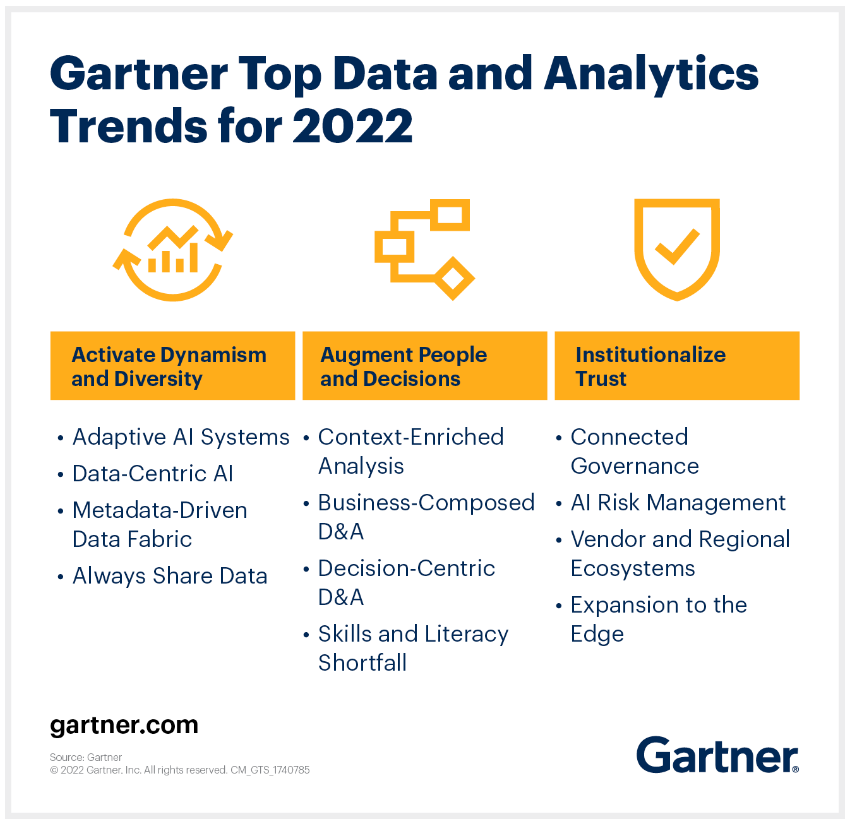
These advancements in the data analysis industry with the integration of AI have made it an essential tool for organizations to stay competitive and make informed decisions.
Applications of AI in Data Analysis
One of the most significant applications of AI in data analysis is predictive modeling.
- Predictive Modeling: Using data from the past to make predictions about the future. This can be used in various scenarios, such as customer behavior analysis, fraud detection, and supply chain management. For example, a retail company can use predictive modeling to predict which products will sell well in the future, and adjust their inventory accordingly.
- Anomaly Detection: Identifying patterns in data that do not conform to expected behavior. This can be used to detect fraud, network intrusion, or equipment failure. For example, a bank can use anomaly detection to identify unusual transactions that may indicate fraudulent activity.
- Image Recognition: Identifying objects, people, or activities in images or videos. This can be used in various scenarios, such as security surveillance, medical imaging, and autonomous vehicles. For instance, a company that provides security cameras can use image recognition to detect individuals who are breaking into the building.
- Recommender Systems: Building systems that can recommend products or services to users based on their preferences or past behavior. This can be used in various scenarios, such as e-commerce and online media platforms.
- Sentiment Analysis: Understanding the emotions, opinions, and attitudes of people towards a particular product or service, this can be used in various scenarios, such as social media monitoring, marketing, and customer service.
- Speech Recognition: Recognizing spoken language and converting it into text. This can be used in various scenarios, such as voice-controlled assistants and call centers.
- Text-to-Speech: Converting written text into spoken language. This can be used in various scenarios, such as virtual assistants and accessibility tools.
- Robotics and Autonomous Systems: Building robots and autonomous systems that can perform tasks without human intervention. This can be used in various scenarios, such as manufacturing, transportation, and healthcare.
- Predictive Maintenance: Predictive maintenance uses data, analytical models, and AI techniques to predict when equipment or machinery is likely to fail, this can be used in various scenarios, such as manufacturing, transportation, and healthcare.
- Fraud Detection: Identifying fraudulent activities by analyzing large amounts of data. This can be used in various scenarios, such as credit card transactions, insurance claims, and tax returns.
- Supply Chain Optimization: Optimizing the flow of goods and services in a supply chain by analyzing data on inventory, transportation, and demand.
- Healthcare: AI-based data analysis is increasingly being used in healthcare to predict disease, improve drug discovery, and streamline healthcare delivery.
- According to a study by Accenture, AI has the potential to create $150 billion in annual savings for the healthcare industry by 2026.
Tools and Technologies
The main tools and technologies used for AI-based data analysis are:
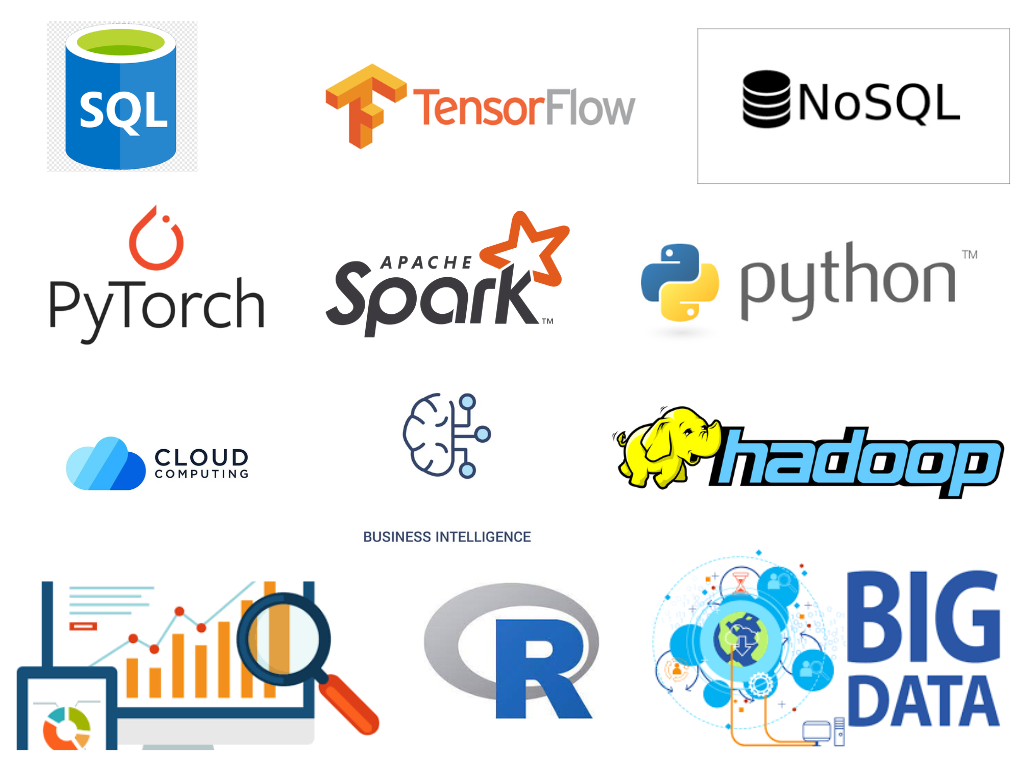
Best Practices
Best practices for using AI in data analysis include data preparation, feature engineering, and model evaluation.
- Data Preparation: Clean and preprocess the data, handling missing values and outliers, to ensure that the data is ready for analysis.
- Feature Engineering: Select and create the right features that will be used in the model, this is a crucial step as it can significantly impact the model’s performance.
- Model Evaluation: Assess the performance of the model and make adjustments as needed, you can use techniques such as cross-validation, and evaluate the model’s performance using metrics such as accuracy, precision, and recall.
- Data Governance: Make sure the data is properly governed, this means ensuring that the data is accurate, consistent, and up-to-date, and that it is protected from unauthorized access.
- Continual Learning: Continuously monitor the performance of the model and make adjustments as needed, this will help to ensure that the model stays up-to-date with the latest trends and patterns in the data.
ChatGPT – Top 5 ChatGPT’s new potential business ideas
Challenges and Limitations
- Data Quality: The quality of data plays a crucial role in the accuracy of AI-driven decision making. Poor quality data can lead to inaccurate conclusions and flawed decisions.
- Data Governance: As AI algorithms process large amounts of data, it’s important to have proper data governance in place to ensure data privacy, security, and compliance.
- Explainability: Some advanced AI algorithms can be difficult to interpret and understand, making it challenging to explain the reasoning behind certain decisions.
- Bias: AI algorithms are only as unbiased as the data they are trained on. If the training data is biased, the AI model will also be biased and can lead to unfair and discriminatory decisions.
- Lack of domain expertise: AI-driven decision making requires a strong understanding of the domain and the problem at hand. Without this expertise, it can be difficult to properly configure the AI algorithms and interpret the results.
- Cost: Implementing and maintaining AI-driven decision-making systems can be costly, especially for small and medium-sized businesses.
- Integration: Integrating AI-driven decision-making systems with existing systems and processes can be challenging and requires a significant investment in time and resources.
- Human oversight: AI systems can make mistakes or fail to understand the context of a situation. It is important to have human oversight to ensure that the AI system is making the right decisions.
Conclusion: The Future of AI in Data Analysis
In conclusion, AI-based data analysis has the potential to revolutionize the way organizations approach data analysis. With the ability to process large amounts of data quickly and accurately, AI can help organizations to extract meaningful insights, make better decisions, and gain a competitive edge.
The integration of AI in data analysis has led to significant advancements in the field and has opened up new possibilities for organizations.
However, it is important for organizations to understand the challenges and limitations that come with using AI for data analysis, such as data bias and interpretability.
As technology continues to evolve, the future of AI in data analysis looks bright, and it is expected to bring even more benefits to organizations.
It is crucial for organizations to stay up-to-date with the latest developments in AI and data analysis to ensure that they can take full advantage of the opportunities that it presents.
Sare your thoughts and ideas in the comments below.
To get in touch with us, please send an email to dataspaceconsulting@gmail.com or contactus@dataspacein.com.
You can also visit our website – DataspaceAI